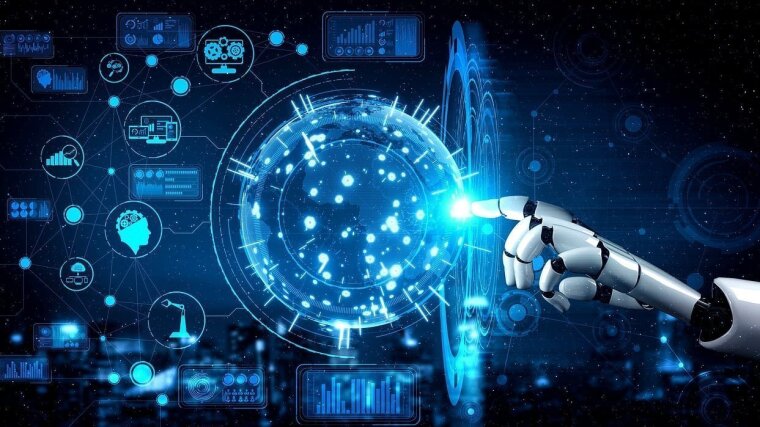
Background
In the last decade, AI technologies have experienced a revolution and have quickly become indispensable tools for countless applications, from everyday life to fundamental scientific research that benefit from the machine learning methods. With the massive spread of AI, new challenges also emerged, particularly regarding the massive energy consumption and the resulting environmental footprint of AI technologies, which could put a restriction on the spread of their utilization and also on the complexity and the speed of the AI operations. To overcome this obstacle in the path of AI technologies, there has been a strong research push towards using physical neuromorphic systems instead of the established classical, computer-based systems. Since photons travel with the speed of light, photonic neuromorphic systems are a highly promising candidate for this challenge. Moreover, since photonic systems can feature very low power consumption, they can overcome some of the main challenges of current AI technologies. As an added bonus, photons have multiple physical degrees of freedom, such as polarization, spatial/modal, and spectral. These multitude of free degrees can be used for carrying and transmitting information, and hence holds the potential to significantly enhance the information processing capacity and density of neuromorphic systems.
Because of these advantages, the last decade has witnessed a surge in research on neuromorphic photonic systems, especially for realizing optical neural networks. Especially, nanostructured integrated and diffractive photonic platforms have been the main ground for this research, as they allow for the realization of compact optical components with highly controlled responses. This brings about the potential of realizing optical neural networks with a massive number of photonic neurons and unparalleled information processing and computational capacities.
At the same time and in parallel, researchers all over the world have contributed to a strong push for the utilization of photonic systems for quantum information processing, e.g. realizing true photonic quantum computers. Today, there are already commercial companies working towards this goal. The hardware and software technologies developed for quantum photonic applications are natural candidates to be adapted for neuromorphic photonic systems. Consequently, this also attracted the interest from the photonic quantum technology community towards neuromorphic systems, and also led to the development of novel ideas such as quantum optical neural networks and quantum machine learning, that seek to use the quantum speedup in neuromorphic systems.
Program committee
- Alexander Breuer (Advanced Computing, University Jena)
- Dmitrii Pidgaiko (Photonic Nanomaterials, University Jena)
- Falk Eilenberger (Micro- and Nanostructured Optics, Fraunhofer Institute for Applied Optics and Precision Engineering Jena, Germany)
- Frank Setzpfandt (Quantum Optics, University Jena)
- Isabelle Staude (Photonic Nanomaterials, University Jena)
- Joachim Giesen (Algorithm Engineering for Machine Learning and AI, University Jena)
- Mario Chemnitz (Smart Photonics, Leibniz Institute for Photonic Technologies Jena)
- Martin Gärttner (Quantum Information, University Jena)
- Sina Saravi (Neuromorphic & Quantum Photonics, University Jena)
- Thomas Pertsch (Nano & Quantum Optics, University Jena)